In the two previous posts on insurance fundamentals, I introduced a fundamental inequality relating payouts to premiums and the concept of risk aversion. Today we will look at this through a bit of a numerical example.
We will look at a risk that has a 1 in 100 chance of occurring and when it does happen the damage it causes is $50. A fair premium rate for this would be 50 cents (1/100 * $50), where fair means that your expected loss is equal to your expected premium payment. Now as we saw due to risk aversion people will be willing to pay a higher than fair rate and still chose to insure themselves.
Now we will work through what the likelihood of the insurance scheme having enough money to cover all losses is assuming that we have 100 people in it. We can use the binomial distribution to find the precise probability of 0, 1, 2, 3 … people experiencing losses. Thanks to the wonderful Wolfram Alpha, here is a plot of what that looks like

So we see that the probability of no loss and exactly 1 loss occurring are each roughly 36% (0.36). The probability of 2 losses occurring is still nearly 20% (0.18) and there is a 6% probability of 3 losses occurring. From there the decline is quite rapid and 4 losses have only a probability of 1.5% and 5 losses are at 0.3%.
What does this mean? Well we can now easily see how many of these loss scenarios are covered at different premium levels.
If people pay exactly the fair premium of 50 cents, then we can cover up to 1 loss, which is 72% of the cases (it includes of course the 36% in which there is no loss at all).
If people are sufficiently risk averse to pay $1 in premium, then we can cover up to 2 losses which is 90% of the cases (36% no loss + 36% 1 loss + 18% 2 losses).
Put differently: even at 2x the premium relative to the “fair” rate we are left with 10% of cases where our insurance scheme does not have enough money in it to pay all the claims!
But we also have 36% of cases in which we have all of our premiums left over because there was no claim at all. This is what creates the possibility of reinsurance. We could go with our scheme to someone else and offer to pay a portion of the premiums we collected in the case that there is no claim or only 1 claim in return for them paying us in case there are more than 2 claims.
This is the point where a textbook would say something like: it is left as an exercise for the reader to verify that there are in fact enough left over premiums to cover the conditional expectation of more than 2 losses through reinsurance (let’s see if anyone takes this bait!).
What has been the underlying assumption of all of the math in this example? That these risks are entirely independent from each other. Meaning in this group of 100 insureds the probability of any one experiencing a loss is not at all correlated with the probability of someone else.
Imagine the extreme example of 100% correlation. Meaning either everyone experiences a loss or no-one. It could still be true that the individual probability of experiencing a loss is just as before 1 in 100. But when it happens it happens to everyone. Can we have our insurance scheme in that case?
Absolutely not. We would be confronted with the strange situation of having paid premiums which don’t go used in 99% of the cases and in 1% case everyone experiences a loss at the same time.
What about somewhere in between? Well with some degree of correlation it means that probability gets shifted into the tail where a larger number of losses occurs simultaneously. So if, for instance, our reinsurance scheme is based on assuming no correlation but there is some, then the reinsurance provider will look very profitable for some time only to get suddenly wiped out entirely.
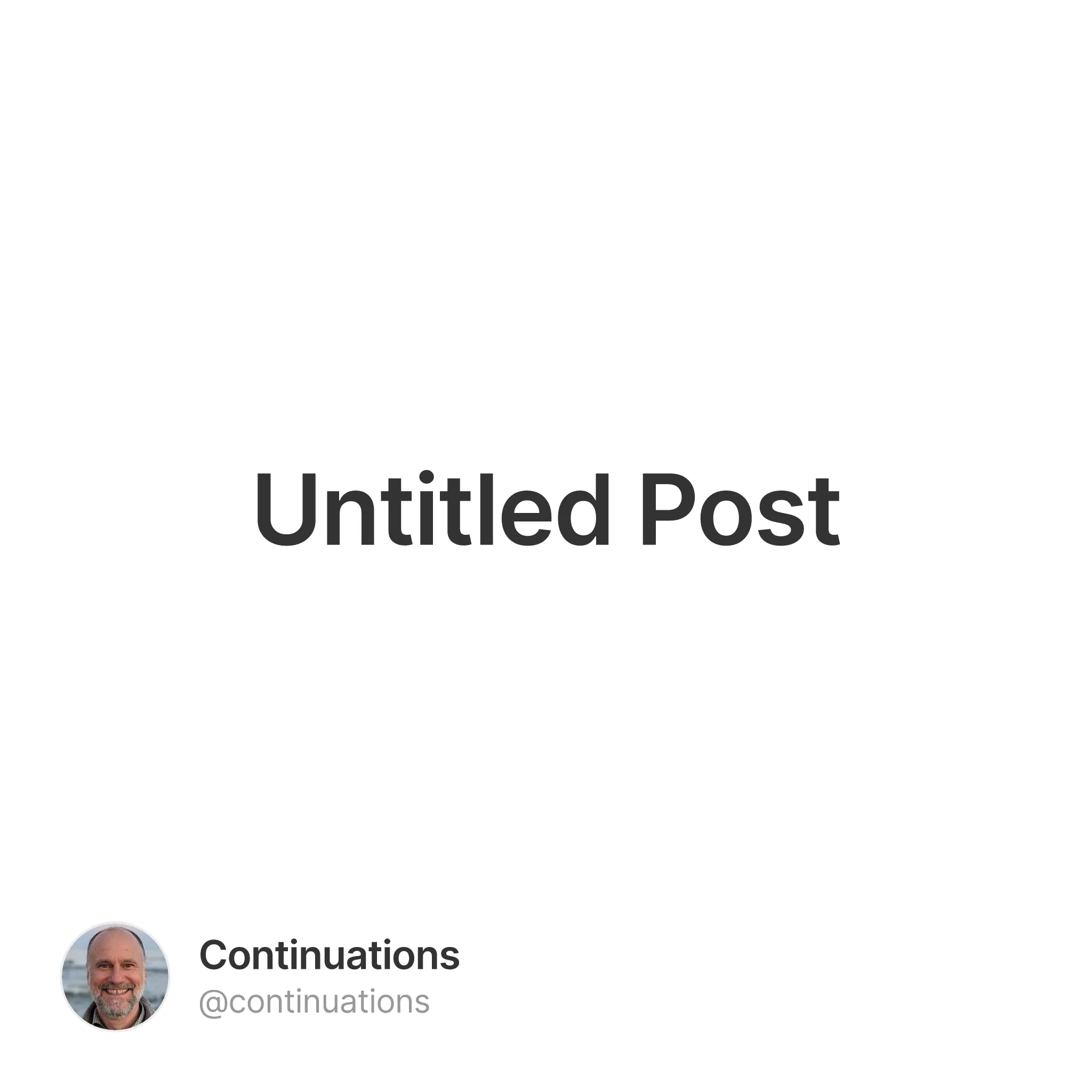
