After a short break of a few weeks, Uncertainty Wednesday is back! My last post had been the third part in a series on “Spurious Correlation” which ended with “Interestingly, this takes me to the edge of my own knowledge and so I have asked an expert in Bayesian estimation to help me. Stay tuned!” Well, that expert is Eric Novik from Generable. Generable is a company that uses Stan, the world’s leading Bayesian estimation too, to help companies make better decisions.
Eric took on my challenge of representing a prior belief about correlation and seeing how observed correlation in a small sample would change that belief. You can find Eric’s complete post, titled “Correlation or no correlation, that is the question” on the Generable blog. The post is quite technical and I will not reproduce it here. Instead, I want to show the key findings in the form of density charts that Eric kindly prepared for me.
Here is the first chart

On the right hand side it shows the prior probability distribution over different correlation values in a so-called violin plot. Relative to some of the pictures I have shown in the past this simply has the axes switched, so on the vertical axis you have the possible correlation values from -1.0 to +1.0 and on the horizontal axis you have the probabilities. Now the picture combines the prior and posterior distributions into one, so you have to imagine on the horizontal axis there is a 0 where the respective words are. The graph is then mirrored around the 0 probability axis to make a nice looking solid shape. The human eye and brain can compare those solid shapes more easily with each other.
What then do we see? Well the green colored prior distribution here has all possible correlation values from -1.0 to +1.0 with roughly the same probability. This corresponds to having no prior belief about a specific correlation being more likely than another correlation. As I pointed out, this is a much more relaxed assumption than what is often assumed instead, namely that correlation = 0, i.e. the variables are uncorrelated.
The blue dotted line shows the correlation of -0.38 that was observed in the specific sample. The red colored distribution is the posterior distribution over possible correlation values. With our very relaxed prior we now see that a lot more probability mass resides close to the observed correlation in the sample, but we also see that lots of other correlation values are still included in the distribution, including positive correlation values up to greater than +0.5.
Now the super cool thing about the approach that Eric took is that we can easily try a different prior (in his code his requires changing a single parameter). Here is a second example:

Before reading on, ask yourself if you can interpret this chart compared to the first chart. What is different about the prior distribution and how does that impact the posterior?
So the prior now has much more probability around correlation = 0. Meaning we belief that the variables are more likely to be uncorrelated, but we are not ruling out either extreme from -1.0 to +1.0 (you can see there is some probability mass on both ends). With this somewhat tighter prior, we find that the posterior moves a lot less! Much more mass remains above the sample correlation and the mean correlation in the posterior (the slightly darker red horizontal line) is about halfway between uncorrelated (0 correlation) and the observe -0.38 correlation from the sample.
What should you take away from all of this? Correlation, like mean, is just a single point statistic. As such it has a distribution of its own. Most people make the mistake of ignoring the existence of that distribution which results in all sorts of errors of inference. They do so either because they never really understood this, or maliciously in what has become known as “p-hacking.” In upcoming posts I will write about p-values and why they are so problematic.
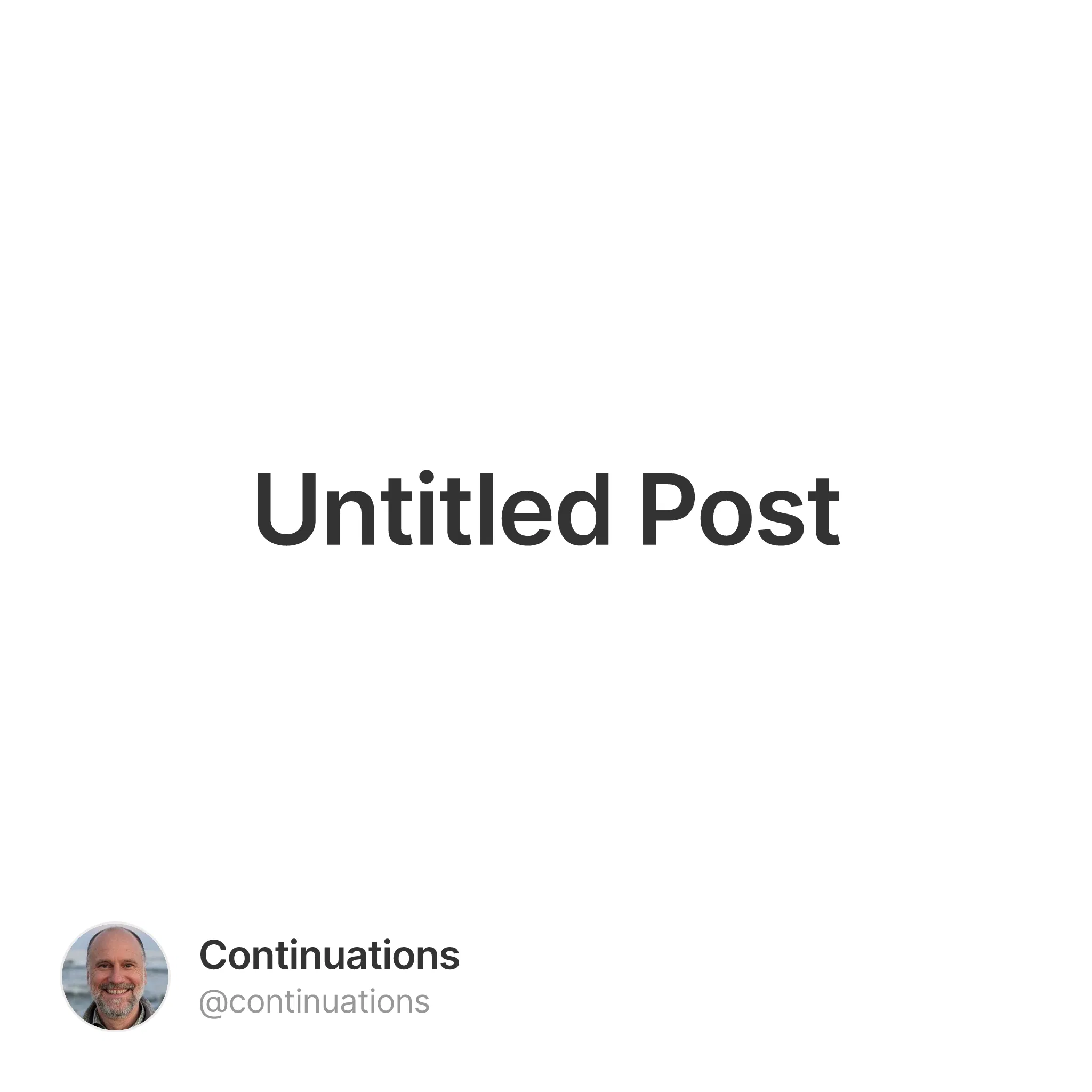
