The last few weeks in Uncertainty Wednesday, with the exception of my net neutrality post, we have been looking at the relationship between sample data and distributions. Today is a bit of a recap so that we know where we are. One of the reasons for writing this series is that in the past I have found that it is super easy to get into lots of detail on mechanics and in the process lose sight of how everything hangs together.
So now is a good time to remind ourselves of the fundamental framework that I laid out early on: we have observations that provide us with signals about an underlying reality. Uncertainty arises because of limitations on how much we can learn about the reality from the observations. We looked at both limitations on the observations and limitations on explanations.
In the posts on samples and how they behave we have been working mostly in the opposite direction. That is we assumed we had perfect knowledge of the underlying reality. For instance, in the first post we assumed we had a fair dice that produced each number from 1 to 6 with exactly probability 1/6. In a later post we assumed we had a perfectly Cauchy distributed process. In each case we then proceeded to produce observations samples *from* that assumption.
Sometimes people call this the study of probability and reserve the term statistics for going the opposite direction, the one we are usually interested in, i.e. from the observations to improved knowledge about the underlying reality. Another term that you will hear in this context is “inference.” We are trying to infer something about reality from the data .
What then should be the key takeaway about inference from the last few weeks? That for some realities we can learn a lot from even relatively small samples, while for others that is not possible. Making this statement more precise will be a big part of Uncertainty Wednesday going forward. But for now you may have an immediate allergic reaction to the implied circularity of the situation. We are trying to learn about reality from observations but we don’t know how much we can learn unless we make assumptions about which reality we are dealing with. Welcome to uncertainty.
How do we cut this circularity? We do so only over time through better explanations. Explanations connect our observations to the reality. We start with a pretty bad explanation, which results in poor inference and a cloudy view of reality. We will then often use that view of reality to make predictions and compare those to future observations (possibly from experiments). Discrepancies arise, which lead us to consider different explanations. Some of those will emerge as better. They make better predictions. They fit better with subsequent observations.
This is why explanations are central to understanding uncertainty (and central to all of science). Too often, however, treatments of uncertainty make all sorts of implicit assumptions. For instance, assumptions of normality or at a minimum of thin tails abound (when we saw that fat tails behave wildly differently). Even when the distribution assumptions are explicit, they are often not related to a specific explanation.
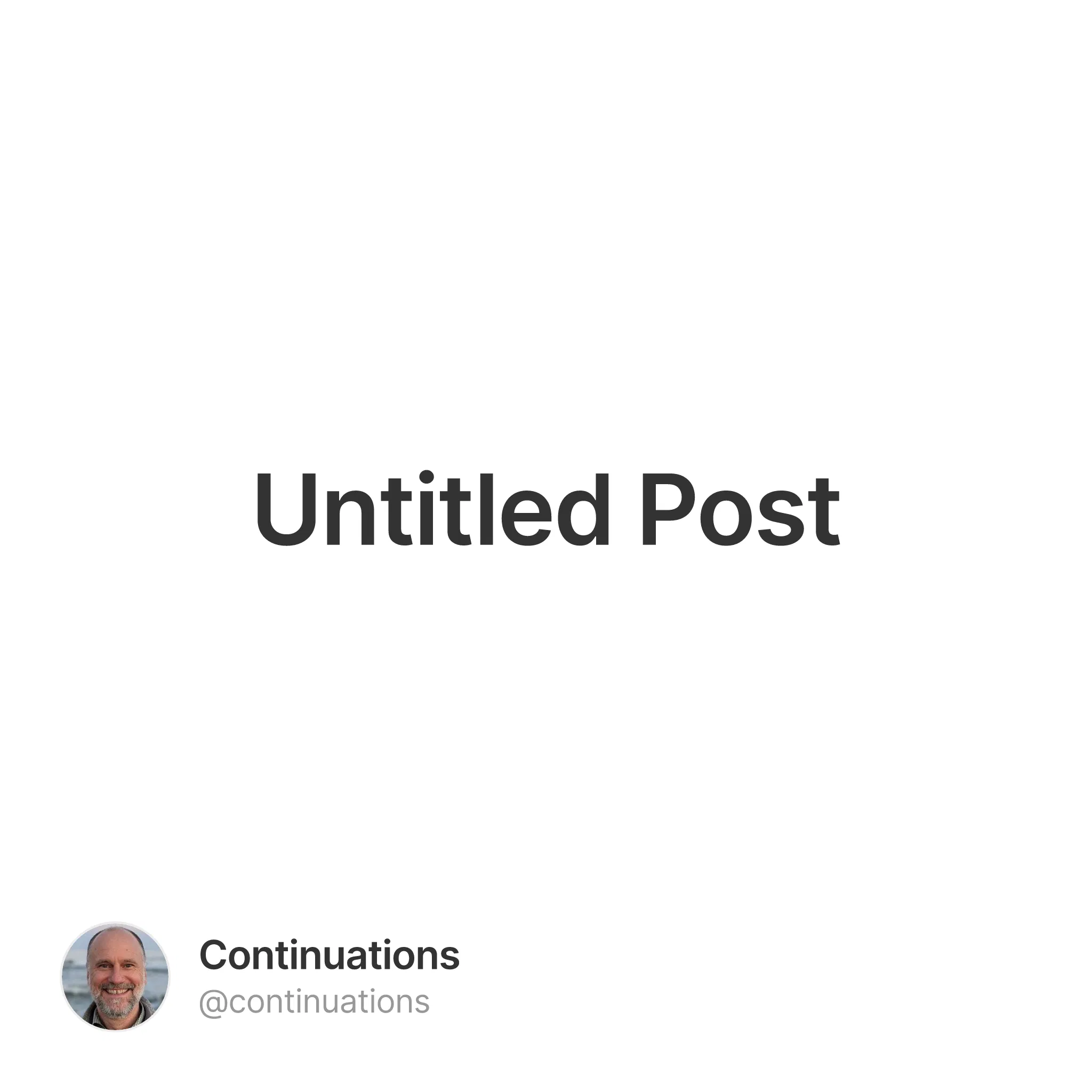
